题目:CG-SSD: Corner Guided Single Stage 3D Object Detection from LiDAR Point Cloud
作者:马瑞琪,陈驰*,杨必胜、李德仁、王海平、丛阳滋、胡宗田
来源:ISPRS Journal of Photogrammetry and Remote Sensing
研究背景:
近年来,随着新能源汽车的不断发展,自动驾驶技术逐渐进入大众视野。其中环境目标感知是自动驾驶的核心技术,得到越来越多的学者的关注。激光LiDAR是自动驾驶车辆中用于目标感知的主要传感器,但由于其获取的点云数据的稀疏性和离散性,相比于传统的图像目标检测面临更多的挑战。因此如何设计高效和高精度的点云3D目标检测模型是一具有意义的科学研究问题。
研究方法:
本文针对LiDAR传感器在获取目标点云时仅能获取部分表面数据的特性,设计了基于角点检测辅助的3D目标检测方法。我们通过对目标点云的分析发现,在BEV(鸟瞰图或俯视图)视角下,LiDAR传感器能够至少扫描到一个目标的角点,但有部分角点是无法被观测到的。因此我们将角点分为可视角点、部分可视角点和不可视角点(图1),并且实验发现可视角点是很容易被检测到,而其余的角点则反之。基于此我们设计网络用于预先检测部分可视角点和不可视角点,通过融合角点的位置信息,为目标中心的检测以及尺寸的回归提供更有利的先验知识。整体的网络模型结构如图2所示,且代码将在近期开源。
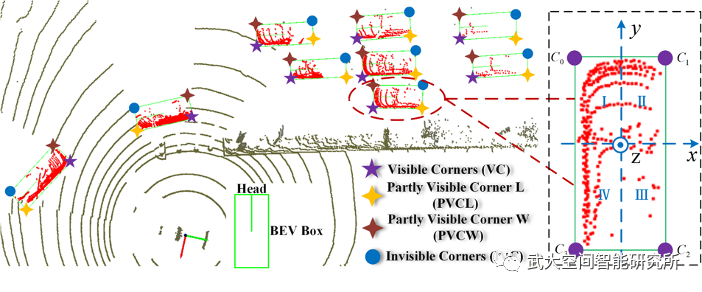
图1 目标角点可视性分类
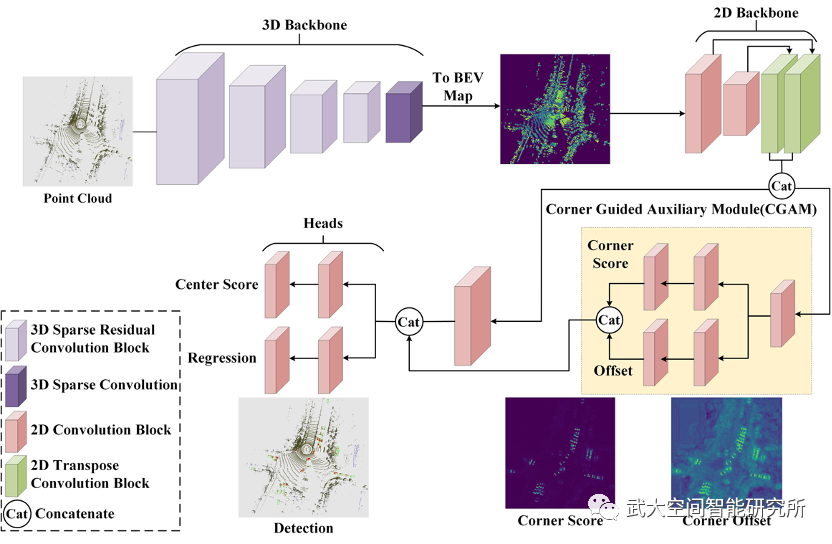
图2 CG-SSD模型结构
研究结果:
在实验结果方面,CG-SSD模型在仅使用单帧点云的情况下,在ONCE数据集达到62.77%mAP,取得当时最好的检测结果,部分可视结果如图3所示。另外,CG-SSD模型中的角点检测模块,可以作为插件的形式作用于绝大多数基于BEV的3D目标检测器,能够带来模型精度的大幅度提升,我们在ONCE和Waymo数据集上的实验结果表面,角点检测增强后的模型相比于原始模型能够提升+1.17%~+14.23% AP。

图3 CG-SSD模型(右)与CenterPoints模型(左)检测结果对比。绿色框为真实值,蓝色框为检测结果。
视频demo
Abstract:
Detecting accurate 3D bounding boxes of the object from point clouds is a major task in autonomous driving perception. At present, the anchor-based or anchor-free models that use LiDAR point clouds for 3D object detection use the center assigner strategy to infer the 3D bounding boxes. However, in the real-world scene, due to the occlusions and the effective detection range of the LiDAR system, only part of the object surface can be covered by the collected point clouds, and there are no measured 3D points corresponding to the physical object center. Obtaining the object by aggregating the incomplete surface point clouds will bring a loss of accuracy in direction and dimension estimation. To address this problem, we propose a corner-guided anchor-free single-stage 3D object detection model (CG-SSD). Firstly, the point clouds within a single frame are assigned to regular 3D grids. 3D sparse convolution backbone network composed of residual layers and sub-manifold sparse convolutional layers are used to construct bird’s eye view (BEV) features for further deeper feature mining by a lite U-shaped network; Secondly, a novel corner-guided auxiliary module (CGAM) with adaptive corner classification algorithm is proposed to incorporate corner supervision signals into the neural network. CGAM is explicitly designed and trained to estimate locations of partially visible and invisible corners to obtain a more accurate object feature representation, especially for small or partial occluded objects; Finally, the deep features from both the backbone networks and CGAM module are concatenated and fed into the head module to predict the classification and 3D bounding boxes of the objects in the scene. The experiments demonstrate that CG-SSD achieves the state-of-art performance on the ONCE benchmark for supervised 3D object detection using single frame point cloud data, with 62.77% mAP. Additionally, the experiments on ONCE and Waymo Open Dataset show that CGAM can be extended to most anchor-based models which use the BEV feature to detect objects, as a plug-in and bring +1.17%~+14.23% AP improvement.