Yue Pan ; Bisheng Yang ; Fuxun Liang ; Zhen Dong
Abstract:
In this paper, we propose a coarse-to-fine integration solution inspired by the classical ICP algorithm, to pairwise 3D point cloud registration with two improvements of hybrid metric spaces (e.g., BSC feature and Euclidean geometry spaces) and globally optimal correspondences matching. First, we detect the keypoints of point clouds and use the Binary Shape Context (BSC) descriptor to encode their local features. Then, we formulate the correspondence matching task as an energy function, which models the global similarity of keypoints on the hybrid spaces of BSC feature and Euclidean geometry. Next, we estimate the globally optimal correspondences through optimizing the energy function by the Kuhn-Munkres algorithm and then calculate the transformation based on the correspondences. Finally, we iteratively refine the transformation between two point clouds by conducting optimal correspondences matching and transformation calculation in a mutually reinforcing manner, to achieve the coarse-to-fine registration under an unified framework. The proposed method is evaluated and compared to several state-of-the-art methods on selected challenging datasets with repetitive, symmetric and incomplete structures. Comprehensive experiments demonstrate that the proposed IGSP algorithm obtains good performance and outperforms the state-of-the-art methods in terms of both rotation and translation errors.
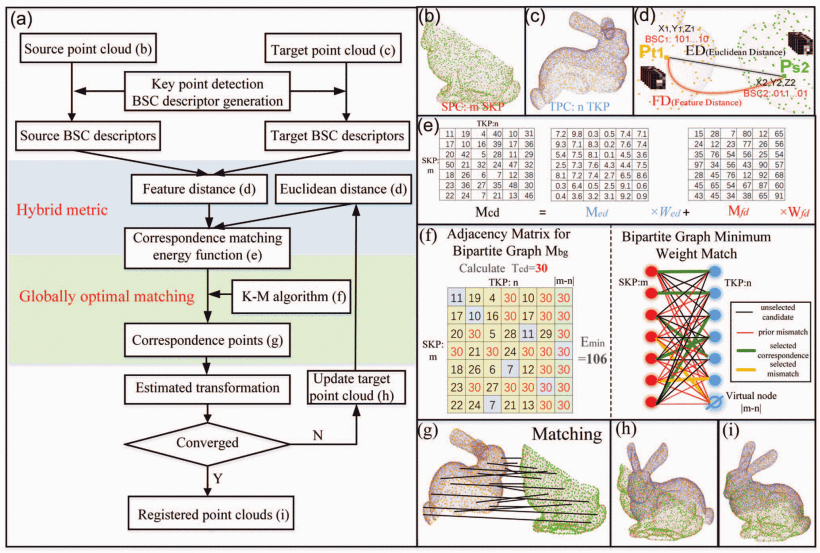

Download