标题:一种用于地面激光扫描快速定位到机载激光扫描点云的天际线上下文描述子
A novel skyline context descriptor for rapid localization of terrestrial laser scans to airborne laser scanning point clouds
作者:Fuxun Liang, Bisheng Yang, Zhen Dong, Ronggang Huang, Yufu Zang, Yue Pan
来源:ISPRS Journal of Photogrammetry and Remote Sensing
机载激光扫描(ALS)能够快速获取观测区域的俯视信息,并通过全球导航卫星系统(GNSS)生成具有全局坐标系的点云。地面激光扫描(TLS)能够收集观测区域的侧视信息,并且站点设置灵活,使其非常适合应用在居住区和街道峡谷等复杂场景中。藉由二者的互补,可以实现对复杂观测区域的三维完整刻画。但是在城市的复杂场景中,常常存在地面GNSS信号不可用的情况,因此,有必要研究一种方法,可以将多个TLS扫描快速自动定位到ALS坐标系下,实现数据的集成。
已有的TLS与ALS数据的集成方法主要包括两种:1)基于局部特征的方法,2)基于全局定位的方法。基于局部特征的方法提取场景中的特征点、线、平面一类的局部特征,并通过恢复同名特征的对应关系来估计变换。在这类方法中,建筑物边界线由于在俯视视角和侧视视角下都具有一定的可见性,因此是最常用的特征。这类方法存在一定的局限性:首先,方法的有效性依赖于提取到的局部特征的数量和质量;其次,局部特征的分布会影响方法的精度;第三,处理大量的ALS数据将非常耗时。
基于全局定位的方法,则是将ALS数据当做观测区域的地图,然后将坐标系间转关关系的求解,转换为TLS在ALS地图中的定位搜索问题。这类方法将匹配问题转换为字典搜索问题,且不要求地面数据各站之间存在重叠,因此更适用于多站的数据处理。
本文提出了一种新的基于天际线上下文描述子的方法,可以将TLS的多站数据快速定位到ALS点云中。天际线上下文描述子考虑了不同视角和分辨率的影响,对场景进行全局的特征编码,并利用天际线的特点加速定位的搜索过程,从而实现快速、准确的自动定位。方法流程如图1。天际线上下文描述子的生成如图2。
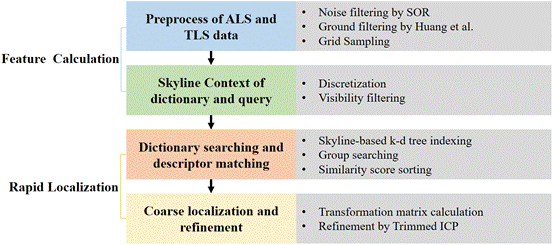
图1 方法流程图
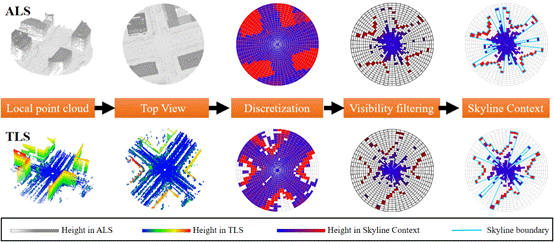
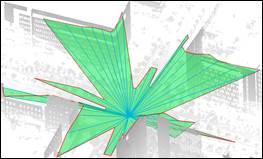
图2 天际线上下文描述子生成示意图
为测试所提方法的有效性,本文选取了5种不同的城市场景数据(住宅区、街道、校园、郊区、高速路),共包含112站地面点云,作为数据集进行实验。部分实验结果如图3、图4。实验结果表明,在使用给定的参数设置时,方法可以实现109站的成功匹配,成功率达到97%,融合结果的最优精度达到0.1米。实验结果的精度评价见表1。
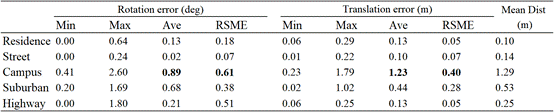
表1 实验结果精度评价
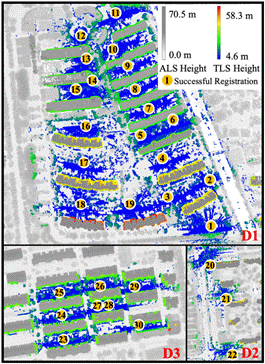
(a) (b)
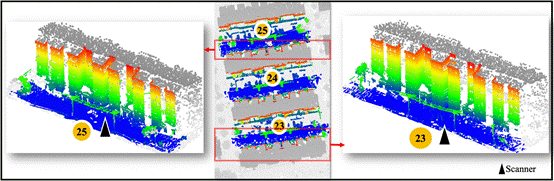
(c)
图3 实验结果及部分细节(住宅区场景,共30站)
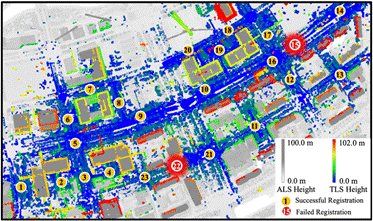
(a) (b)
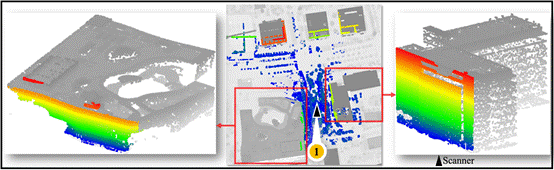
(c)
图4 实验结果及部分细节(街道场景,共23站)
Abstract:
By utilizing the Airborne Laser Scanning (ALS) and Terrestrial Laser Scanning (TLS), the land surface information from both top view and side view can be captured rapidly. However, due to the different perspective views, resolutions, and ranges, the automatic localization of multiple TLS scans to ALS is challenging. To better address this issue, this paper proposes a novel skyline context-based method. First, the ground points in ALS are extracted and used as potential TLS locations, with the corresponding skyline contexts generated. After that, a 3D skyline-based k-d tree indexing is carried out to facilitate searching the corresponding coarse localizations of TLS scans. The final refinement is done by the trimmed iterative closest point algorithm (T-ICP). In order to evaluate the performance of the proposed method, 5 datasets with different ALS sizes and over one hundred TLS scans are adopted to conduct a thorough evaluation. For one ALS data with mean point distance of 0.1 meter, the average localization accuracy reached about 0.13 meter. The experimental results indicate that the proposed method performs well for automatic localization of TLS scans to ALS point clouds, with advantages in both precision and adaptability.