原文题目:Street-view imagery guided street furniture inventory from mobile laser scanning point clouds
ISPRS Journal of Photogrammetry and Remote Sensing
周雨舟,韩旭,彭明军,李海亭,杨波,董震*,杨必胜*
背景
利用车载移动测量系统采集的点云和影像准确提取道路附属设施,掌握其部件级的类别、位置、尺寸、形状等信息,不仅对城市管理和交通规划有重要意义,也是助力智能交通发展和实景三维中国建设的关键因素。但是,现有的单独依赖影像或点云的提取方法难以实现语义、纹理信息和几何、形状信息的平衡,而融合点云和影像的方法在点云实例分割和多视角信息融合等方面仍存在不足,无法满足复杂城市场景中的道路附属设施三维部件级提取要求。
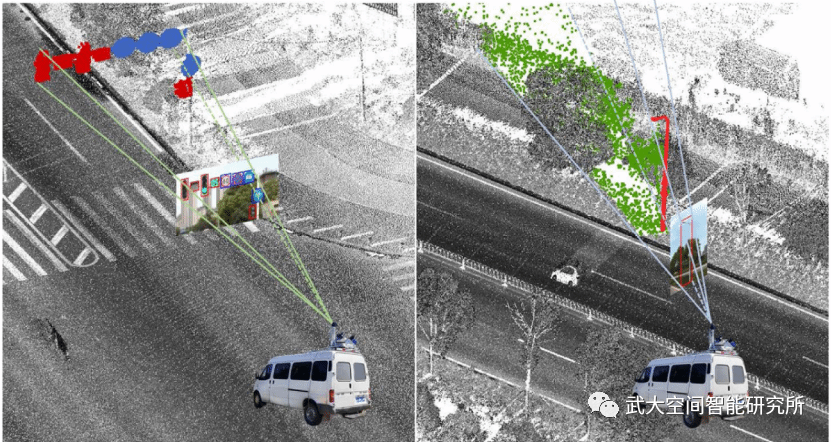
点云-影像融合的道路附属设施提取
方法概述
本文提出一种融合点云和影像的道路附属设施部件级三维提取方法,输出多类别的带有语义标签的道路附属设施准确位置和实例点云。该方法包含以下三个模块:
(1)以街景影像目标检测为指导,根据点云和影像配准关系,裁切道路附属设施视锥点云;
(2)提出联合了实例三维包围盒预测、实例掩码预测和语义分割等多任务的深度学习神经网络进行视锥点云实例分割,输出每个视锥对应的实例包围盒和实例掩码信息;
(3)利用多目标追踪方法建立相同实例多视角视锥关联,在此基础上利用耦合多视角信息的神经网络,进行道路附属设施目标实例精化。
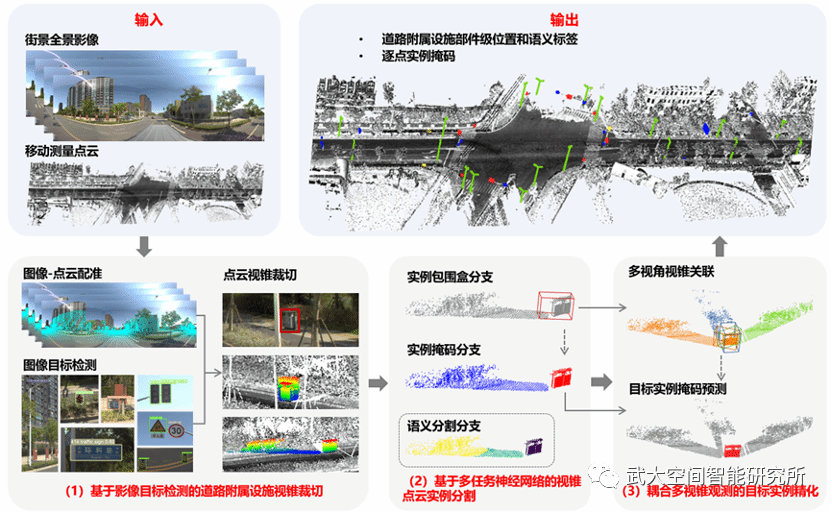
整体技术框架
提取效果
本文使用采集于上海市和武汉市的车载移动测量数据集测试了方法效果。实验表明,该算法的实际应用效果达到了设计目标,采用统一的方法设计和参数设置,对九个类别的典型道路附属设施进行了准确提取。提取结果在上海市数据集的实例级平均召回率和精确率分别为86.4%,80.9%,在武汉市数据集的分别为83.2%,87.8%,在两个数据集的逐点级平均召回率、精确率都超过了73.7%。

上海市数据集提取结果
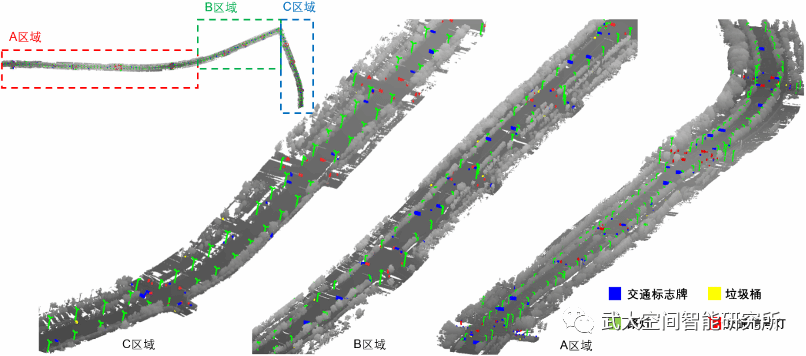
武汉市数据集提取结果
使用该方法可以在城市道路和十字路口处定位道路附属设施,并且输出实例点云,为交通和城市管理提供包括语义类别和位置坐标在内的准确详细的道路资产清查结果,以及包括形状和尺寸在内的三维几何特征。这些道路附属设施不仅是城市交通规划的关键资产,也是自动驾驶高精地图制作的关键提取目标。
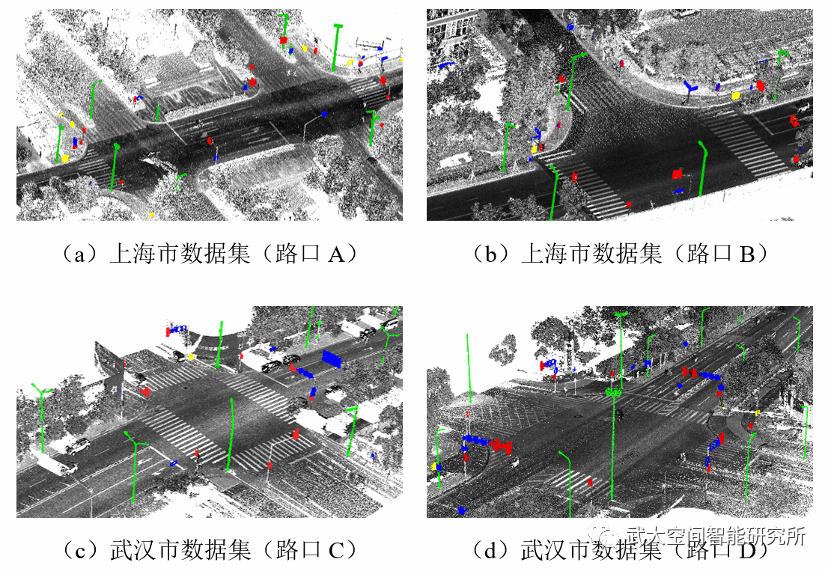
城市路口处提取结果
利用传统的点云目标检测或语义实例分割方法往往难以提取这些小型道路附属设施部件,本文设计了融合点云和影像的提取方法,首先在图像中检测到目标,再在点云中搜索目标实例,有效缩小了搜索空间,提升了对小目标的提取能力。下图展示了锥桶、消防栓、花坛、座椅等小型道路附属设施提取结果。
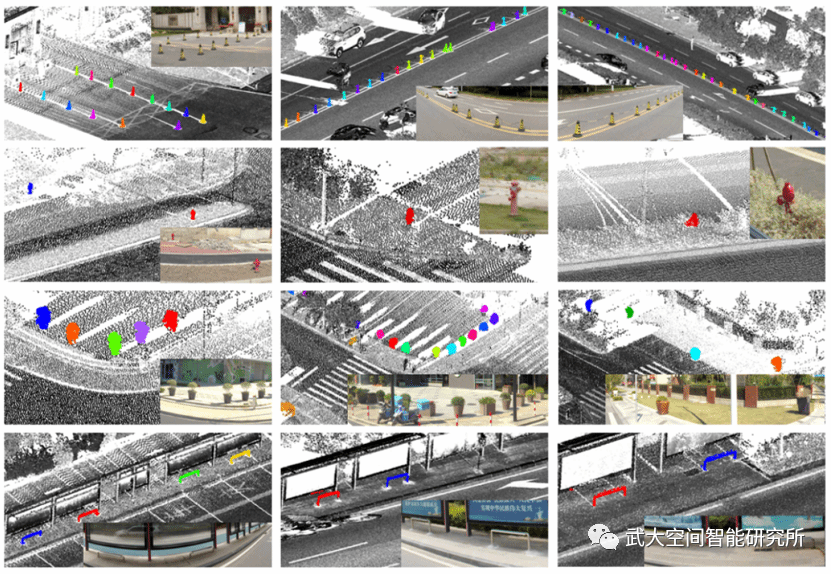
小型道路附属设施提取结果
Abstract
Outdated or sketchy inventory of street furniture may misguide the planners on the renovation and upgrade of transportation infrastructures, thus posing potential threats to traffic safety. Previous studies have taken their steps using point clouds or street-view imagery (SVI) for street furniture inventory, but there remains a gap to balance semantic richness, localization accuracy and working efficiency. Therefore, this paper proposes an effective pipeline that combines SVI and point clouds for the inventory of street furniture. The proposed pipeline encompasses three steps: (1) Off-the-shelf street furniture detection models are applied on SVI for generating two-dimensional (2D) proposals and then three-dimensional (3D) point cloud frustums are accordingly cropped; (2) The instance mask and the instance 3D bounding box are predicted for each frustum using a multi-task neural network; (3) Frustums from adjacent perspectives are associated and fused via multi-object tracking, after which the object-centric instance segmentation outputs the final street furniture with 3D locations and semantic labels. This pipeline was validated on datasets collected in Shanghai and Wuhan, producing component-level street furniture inventory of nine classes. The instance-level mean recall and precision reach 86.4%, 80.9% and 83.2%, 87.8% respectively in Shanghai and Wuhan, and the point-level mean recall, precision, weighted coverage all exceed 73.7%.